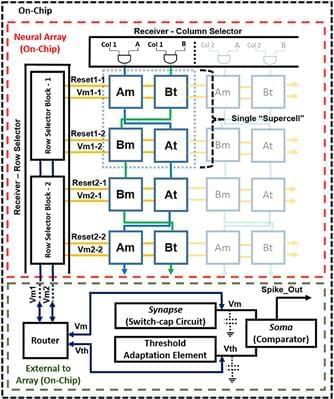
And this feature distinguishes neuromorphic systems from conventional computing systems. The brain has evolved over billions of years to solve difficult engineering problems by using efficient, parallel, low-power computation. The goal of NE is to design systems capable of brain-like computation. Numerous large-scale neuromorphic projects have emerged recently. This interdisciplinary field was listed among the top 10 technology breakthroughs of 2014 by the MIT Technology Review and among the top 10 emerging technologies of 2015 by the World Economic Forum. NE has two-way goals: one, a scientific goal to understand the computational properties of biological neural systems by using models implemented in integrated circuits (ICs); second, an engineering goal to exploit the known properties of biological systems to design and implement efficient devices for engineering applications. Building hardware neural emulators can be extremely useful for simulating large-scale neural models to explain how intelligent behavior arises in the brain. The principal advantages of neuromorphic emulators are that they are highly energy efficient, parallel and distributed, and require a small silicon area. Thus, compared to conventional CPUs, these neuromorphic emulators are beneficial in many engineering applications such as for the porting of deep learning algorithms for various recognitions tasks. In this review article, we describe some of the most significant neuromorphic spiking emulators, compare the different architectures and approaches used by them, illustrate their advantages and drawbacks, and highlight the capabilities that each can deliver to neural modelers. This article focuses on the discussion of large-scale emulators and is a continuation of a previous review of various neural and synapse circuits (Indiveri et al., 2011). We also explore applications where these emulators have been used and discuss some of their promising future applications.
“Building a vast digital simulation of the brain could transform neuroscience and medicine and reveal new ways of making more powerful computers” (Markram et al., 2011). The human brain is by far the most computationally complex, efficient, and robust computing system operating under low-power and small-size constraints. It utilizes over 100 billion neurons and 100 trillion synapses for achieving these specifications. Even the existing supercomputing platforms are unable to demonstrate full cortex simulation in real-time with the complex detailed neuron models. For example, for mouse-scale (2.5 × 106 neurons) cortical simulations, a personal computer uses 40,000 times more power but runs 9,000 times slower than a mouse brain (Eliasmith et al., 2012). The simulation of a human-scale cortical model (2 × 1010 neurons), which is the goal of the Human Brain Project, is projected to require an exascale supercomputer (1018 flops) and as much power as a quarter-million households (0.5 GW).
The electronics industry is seeking solutions that will enable computers to handle the enormous increase in data processing requirements. Neuromorphic computing is an alternative solution that is inspired by the computational capabilities of the brain. The observation that the brain operates on analog principles of the physics of neural computation that are fundamentally different from digital principles in traditional computing has initiated investigations in the field of neuromorphic engineering (NE) (Mead, 1989a). Silicon neurons are hybrid analog/digital very-large-scale integrated (VLSI) circuits that emulate the electrophysiological behavior of real neurons and synapses. Neural networks using silicon neurons can be emulated directly in hardware rather than being limited to simulations on a general-purpose computer. Such hardware emulations are much more energy efficient than computer simulations, and thus suitable for real-time, large-scale neural emulations.