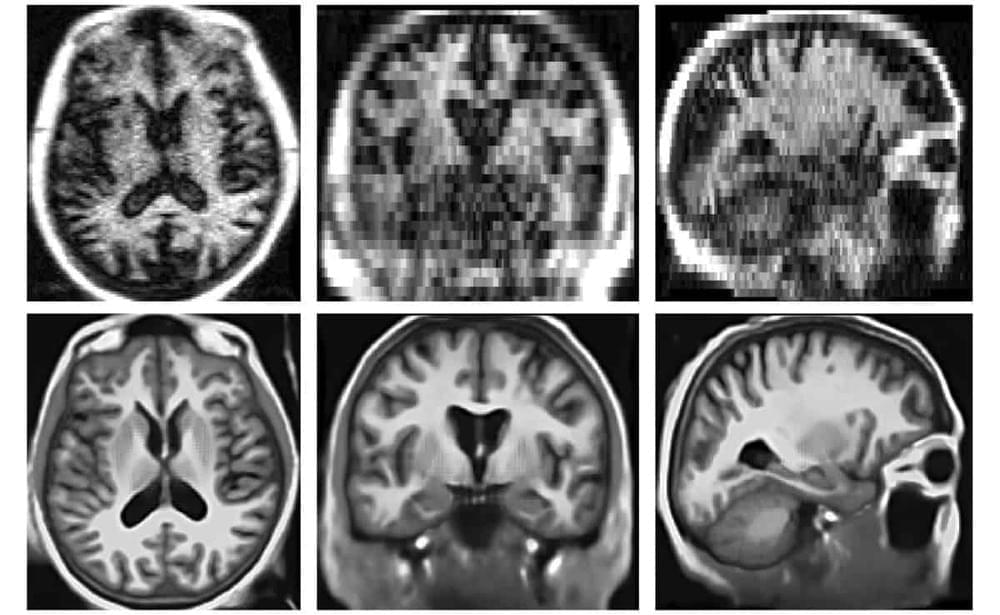
Portable, low-field strength MRI systems have the potential to transform neuroimaging – provided that their low spatial resolution and low signal-to-noise (SNR) ratio can be overcome. Researchers at Harvard Medical School are harnessing artificial intelligence (AI) to achieve this goal. They have developed a machine learning super-resolution algorithm that generates synthetic images with high spatial resolution from lower resolution brain MRI scans.
The convolutional neural network (CNN) algorithm, known as LF-SynthSR, converts low-field strength (0.064 T) T1-and T2-weighted brain MRI sequences into isotropic images with 1 mm spatial resolution and the appearance of a T1-weighted magnetization-prepared rapid gradient-echo (MP-RAGE) acquisition. Describing their proof-of-concept study in Radiology, the researchers report that the synthetic images exhibited high correlation with images acquired by 1.5 T and 3.0 T MRI scanners.
Morphometry, the quantitative size and shape analysis of structures in an image, is central to many neuroimaging studies. Unfortunately, most MRI analysis tools are designed for near-isotropic, high-resolution acquisitions and typically require T1-weighted images such as MP-RAGE. Their performance often drops rapidly as voxel size and anisotropy increase. As the vast majority of existing clinical MRI scans are highly anisotropic, they cannot be reliably analysed with existing tools.