Inspired by biological cells, the robots are made of water encased in Teflon armor and are each about the size of a grain of rice.
Category: robotics/AI – Page 121
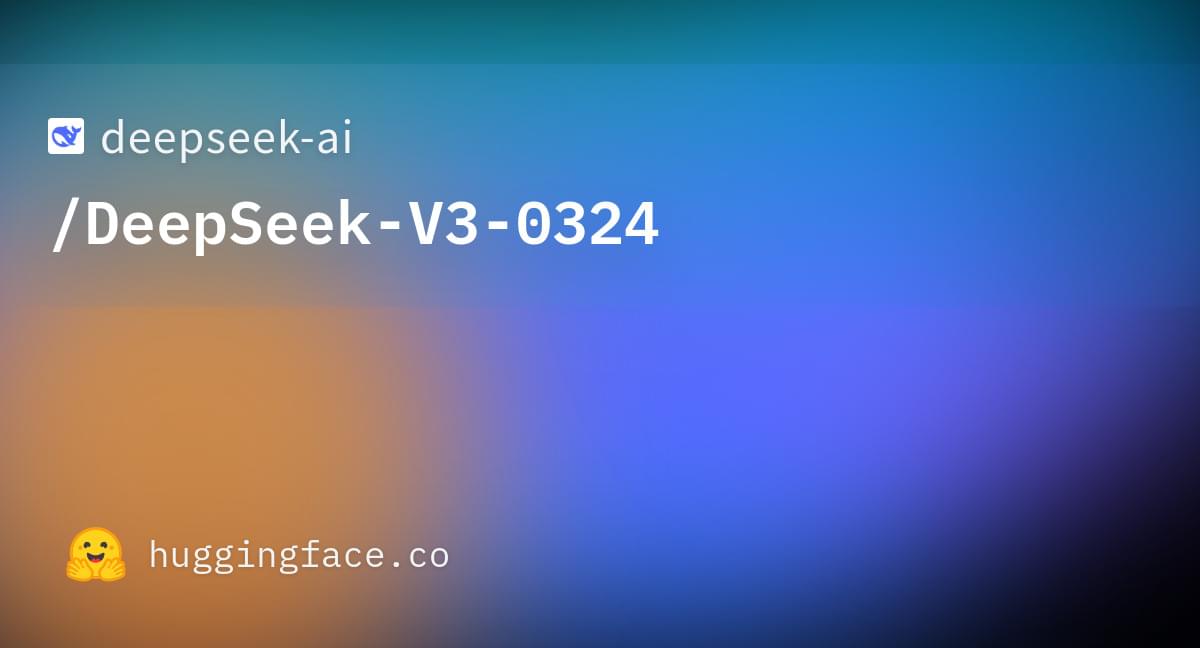
Superconductivity fine-tuned for quantum tech by twisting novel ‘knob’
Researchers have created a tiny, shape-shifting robot that swims, crawls, and glides freely in the deep sea.
Developed by a team at the Beihang University in China, the robot operated at a depth of 10,600 meters in the Mariana Trench.
Using the same actuator technology, a soft gripper mounted on a submersible’s rigid arm successfully retrieved sea urchins and starfish from the South China Sea, demonstrating its capability for deep-sea exploration and specimen collection.
The Next AI Battleground: Why China’s Manus Could Leapfrog Western Agent Technology
China’s Manus AI represents a significant leap from chatbots to autonomous agents capable of executing real-world tasks with minimal human oversight.
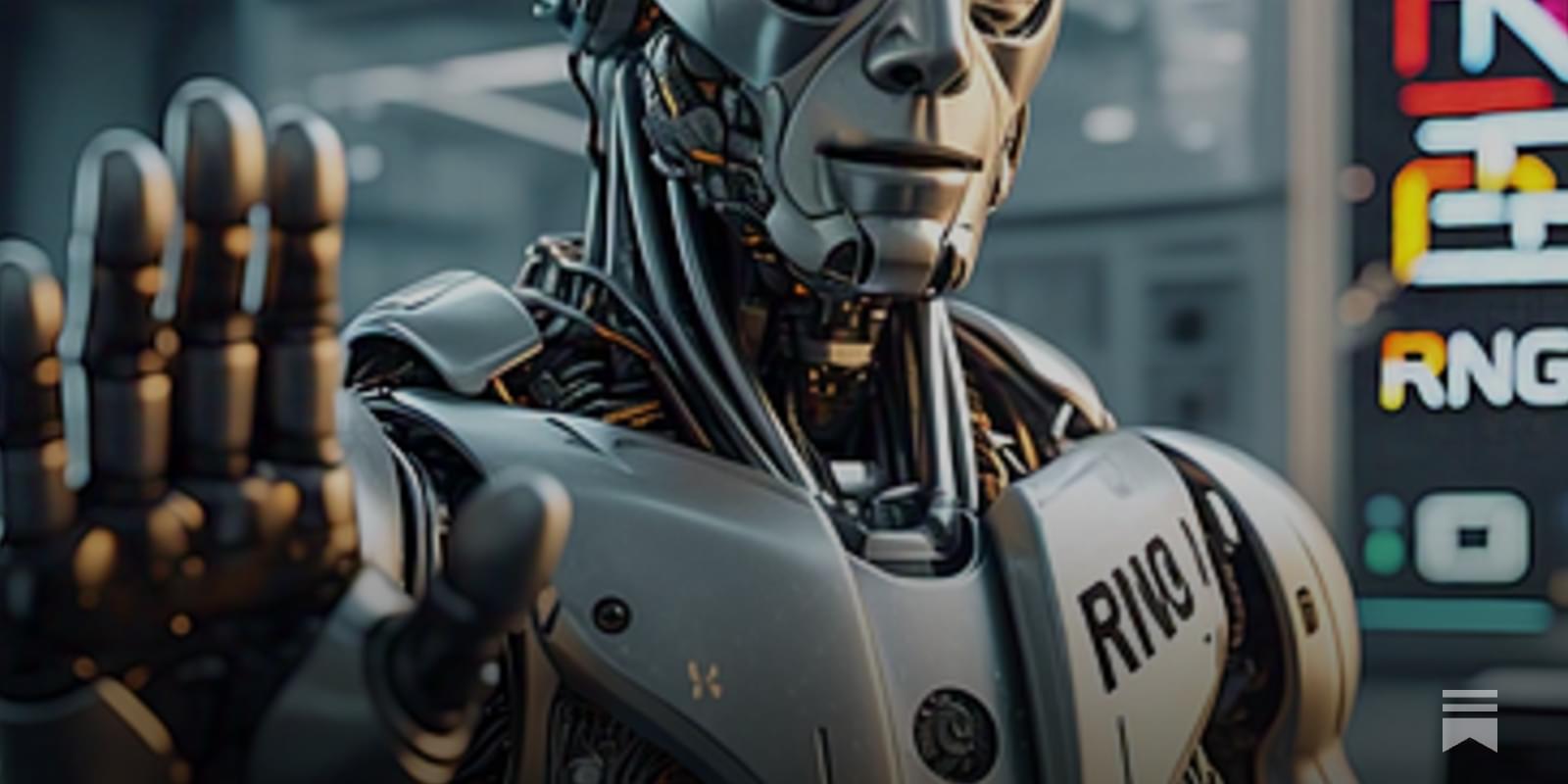
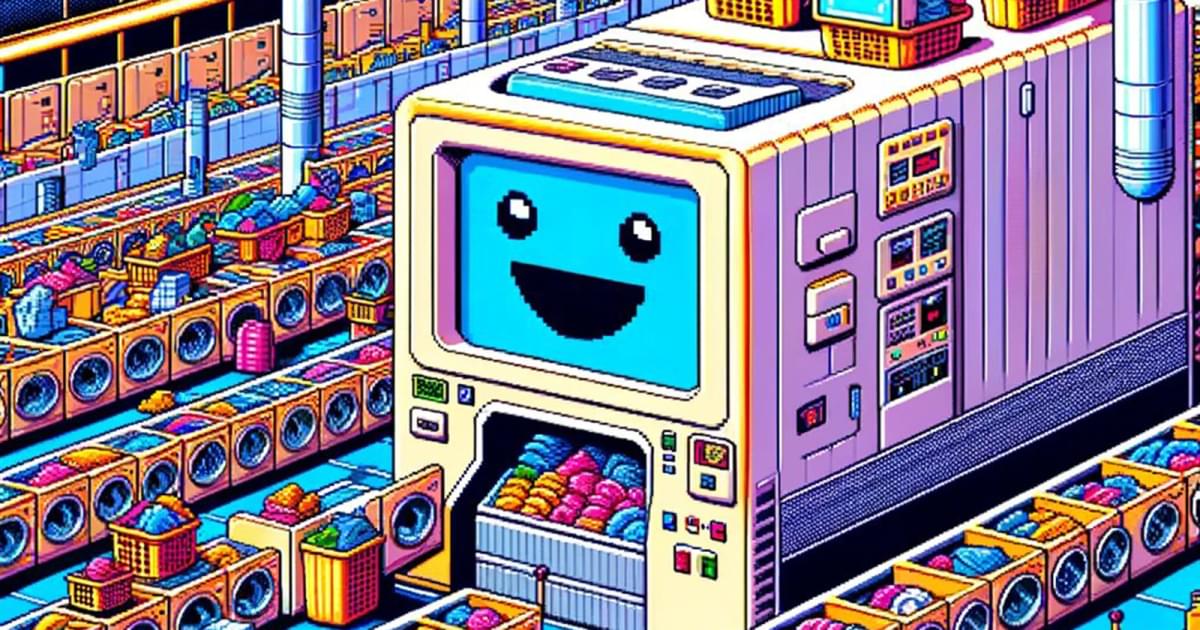
Joscha Bach and Anthony Aguirre on Digital Physics and Moving Towards Beneficial Futures
Joscha Bach, Cognitive Scientist and AI researcher, as well as Anthony Aguirre, UCSC Professor of Physics, join us to explore the world through the lens of computation and the difficulties we face on the way to beneficial futures.
Topics discussed in this episode include:
-Understanding the universe through digital physics.
–How human consciousness operates and is structured.
–The path to aligned AGI and bottlenecks to beneficial futures.
–Incentive structures and collective coordination.
Find the page for this podcast here: https://futureoflife.org/2021/03/31/j… to be the FLI Podcast Producer here: https://futureoflife.org/job-postings/ Follow the podcast on: Spotify: https://open.spotify.com/show/2Op1WO3… Apple Podcasts: https://podcasts.apple.com/us/podcast… SoundCloud: / futureoflife Have any feedback about the podcast? You can share your thoughts here: www.surveymonkey.com/r/DRBFZCT Timestamps: 0:00 Intro 1:58 What is truth and knowledge? 11:39 What is subjectivity and objectivity? 15:13 What is the universe ultimately? 20:32 Is the universe a cellular automaton? Is the universe ultimately digital or analogue? 25:59 Hilbert’s hotel from the point of view of computation 39:14 Seeing the world as a fractal 43:00 Describing human consciousness 57:46 Meaning, purpose, and harvesting negentropy 1:02:30 The path to aligned AGI 1:05:13 Bottlenecks to beneficial futures and existential security 1:16:01 A future with one, several, or many AGI systems? How do we maintain appropriate incentive structures? 1:30:39 Non-duality and collective coordination 1:34:16 What difficulties are there for an idealist worldview that involves computation? 1:37:19 Which features of mind and consciousness are necessarily coupled and which aren’t? 1:47:47 Joscha’s final thoughts on AGI This podcast is possible because of the support of listeners like you. If you found this conversation to be meaningful or valuable, consider supporting it directly by donating at: https://futureoflife.org/donate Contributions like yours make these conversations possible.
Apply to be the FLI Podcast Producer here: https://futureoflife.org/job-postings/
Follow the podcast on:
Joscha Bach: Time, Simulation Hypothesis, Existence
Joscha Bach is a cognitive scientist focusing on cognitive architectures, consciousness, models of mental representation, emotion, motivation and sociality.
Patreon: / curtjaimungal.
Crypto: https://tinyurl.com/cryptoTOE
PayPal: https://tinyurl.com/paypalTOE
Twitter: / toewithcurt.
Discord Invite: / discord.
iTunes: https://podcasts.apple.com/ca/podcast… https://pdora.co/33b9lfP Spotify: https://open.spotify.com/show/4gL14b9… Subreddit r/TheoriesOfEverything: / theoriesofeverything Merch: https://tinyurl.com/TOEmerch 0:00:00 Introduction 0:00:17 Bach’s work ethic / daily routine 0:01:35 What is your definition of truth? 0:04:41 Nature’s substratum is a “quantum graph”? 0:06:25 Mathematics as the descriptor of all language 0:13:52 Why is constructivist mathematics “real”? What’s the definition of “real”? 0:17:06 What does it mean to “exist”? Does “pi” exist? 0:20:14 The mystery of something vs. nothing. Existence is the default. 0:21:11 Bach’s model vs. the multiverse 0:26:51 Is the universe deterministic 0:28:23 What determines the initial conditions, as well as the rules? 0:30:55 What is time? Is time fundamental? 0:34:21 What’s the optimal algorithm for finding truth? 0:40:40 Are the fundamental laws of physics ultimately “simple”? 0:50:17 The relationship between art and the artist’s cost function 0:54:02 Ideas are stories, being directed by intuitions 0:58:00 Society has a minimal role in training your intuitions 0:59:24 Why does art benefit from a repressive government? 1:04:01 A market case for civil rights 1:06:40 Fascism vs communism 1:10:50 Bach’s “control / attention / reflective recall” model 1:13:32 What’s more fundamental… Consciousness or attention? 1:16:02 The Chinese Room Experiment 1:25:22 Is understanding predicated on consciousness? 1:26:22 Integrated Information Theory of consciousness (IIT) 1:30:15 Donald Hoffman’s theory of consciousness 1:32:40 Douglas Hofstadter’s “strange loop” theory of consciousness 1:34:10 Holonomic Brain theory of consciousness 1:34:42 Daniel Dennett’s theory of consciousness 1:36:57 Sensorimotor theory of consciousness (embodied cognition) 1:44:39 What is intelligence? 1:45:08 Intelligence vs. consciousness 1:46:36 Where does Free Will come into play, in Bach’s model? 1:48:46 The opposite of free will can lead to, or feel like, addiction 1:51:48 Changing your identity to effectively live forever 1:59:13 Depersonalization disorder as a result of conceiving of your “self” as illusory 2:02:25 Dealing with a fear of loss of control 2:05:00 What about heart and conscience? 2:07:28 How to test / falsify Bach’s model of consciousness 2:13:46 How has Bach’s model changed in the past few years? 2:14:41 Why Bach doesn’t practice Lucid Dreaming anymore 2:15:33 Dreams and GAN’s (a machine learning framework) 2:18:08 If dreams are for helping us learn, why don’t we consciously remember our dreams 2:19:58 Are dreams “real”? Is all of reality a dream? 2:20:39 How do you practically change your experience to be most positive / helpful? 2:23:56 What’s more important than survival? What’s worth dying for? 2:28:27 Bach’s identity 2:29:44 Is there anything objectively wrong with hating humanity? 2:30:31 Practical Platonism 2:33:00 What “God” is 2:36:24 Gods are as real as you, Bach claims 2:37:44 What “prayer” is, and why it works 2:41:06 Our society has lost its future and thus our culture 2:43:24 What does Bach disagree with Jordan Peterson about? 2:47:16 The millennials are the first generation that’s authoritarian since WW2 2:48:31 Bach’s views on the “social justice” movement 2:51:29 Universal Basic Income as an answer to social inequality, or General Artificial Intelligence? 2:57:39 Nested hierarchy of “I“s (the conflicts within ourselves) 2:59:22 In the USA, innovation is “cheating” (for the most part) 3:02:27 Activists are usually operating on false information 3:03:04 Bach’s Marxist roots and lessons to his former self 3:08:45 BONUS BIT: On societies problems.
Pandora: https://pdora.co/33b9lfP
Spotify: https://open.spotify.com/show/4gL14b9…
Subreddit r/TheoriesOfEverything: / theoriesofeverything.
Merch: https://tinyurl.com/TOEmerch.
0:00:00 Introduction.
0:00:17 Bach’s work ethic / daily routine.
0:01:35 What is your definition of truth?
0:04:41 Nature’s substratum is a \.
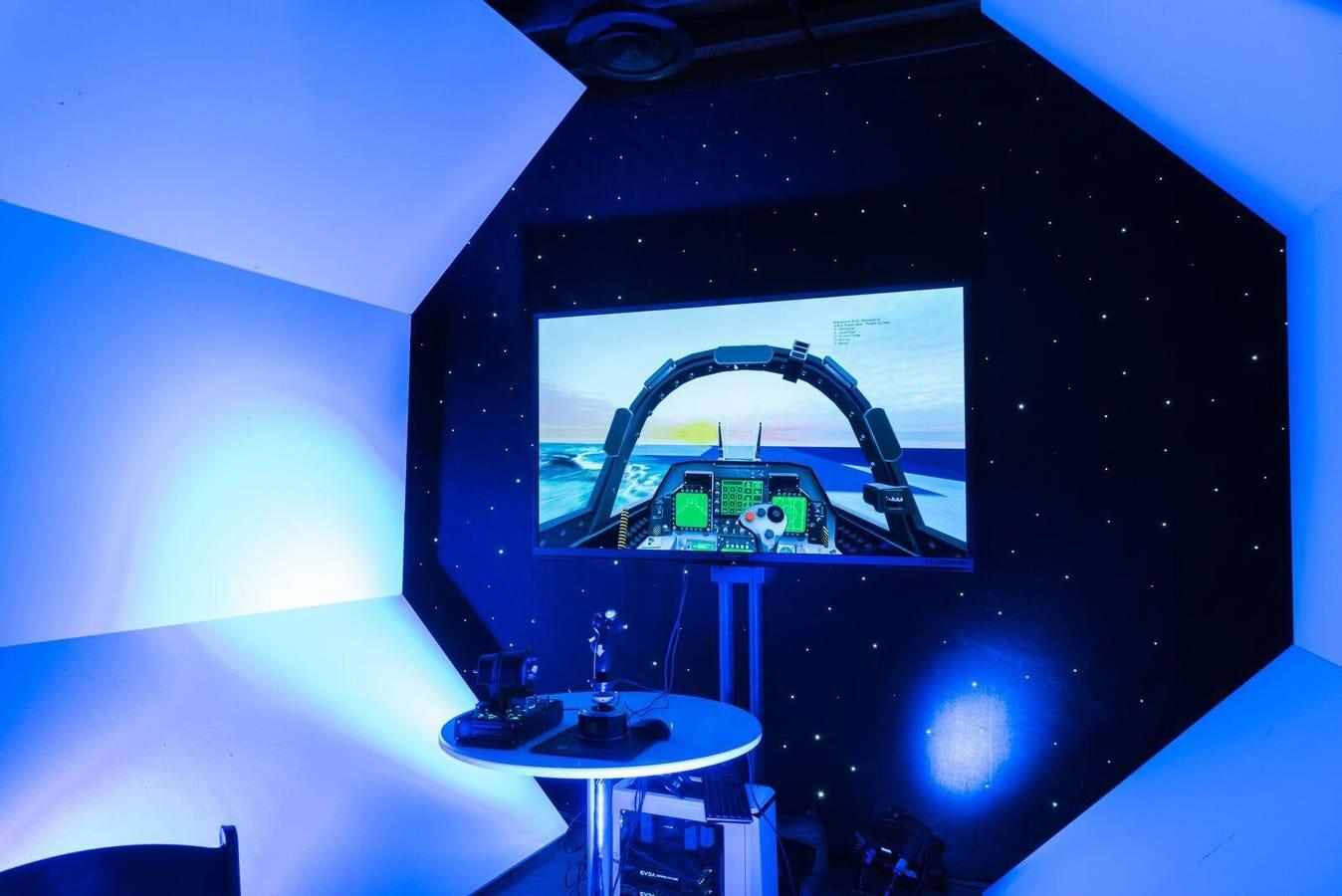