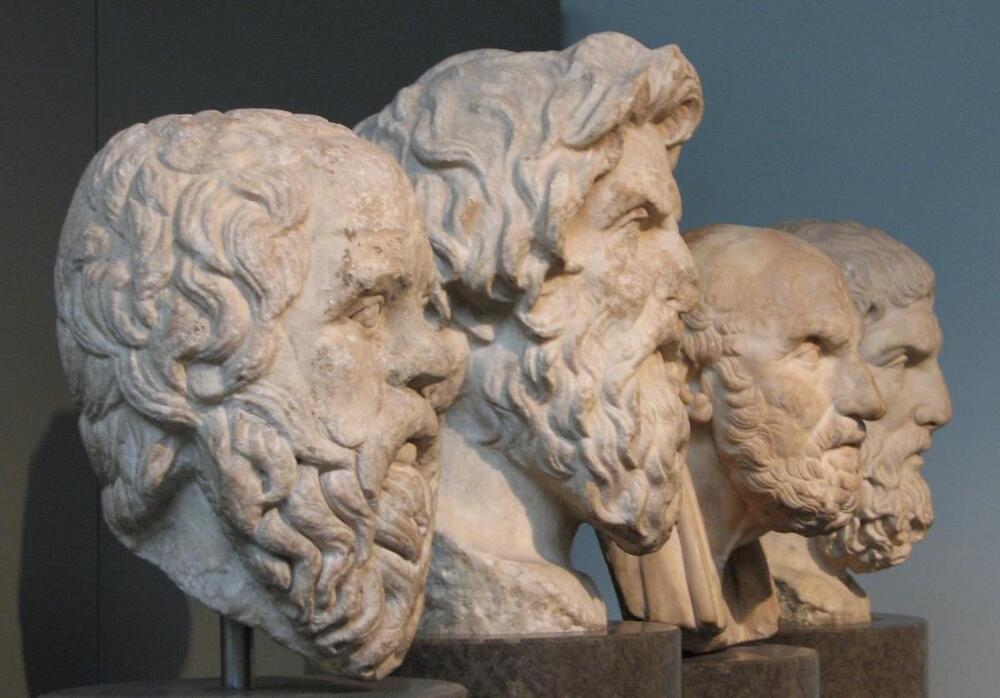
🤖 🏛️ Have you ever wondered about the connection between AI and Ancient Greek Philosophy?
🧔 📜 The ancient Greek philosophers, such as Aristotle, Plato, Socrates, Democritus, Epicurus and Heraclitus explored the nature of intelligence and consciousness thousands of years ago, and their ideas are still relevant today in the age of AI.
🧠 📚 Aristotle believed that there are different levels of intelligence, ranging from inanimate objects to human beings, with each level having a distinct form of intelligence. In the context of AI, this idea raises questions about the nature of machine intelligence and where it falls in the spectrum of intelligence. Meanwhile, Plato believed that knowledge is innate and can be discovered through reason and contemplation. This view has implications for AI, as it suggests that a machine could potentially have access to all knowledge, but it may not necessarily understand it in the same way that a human would.