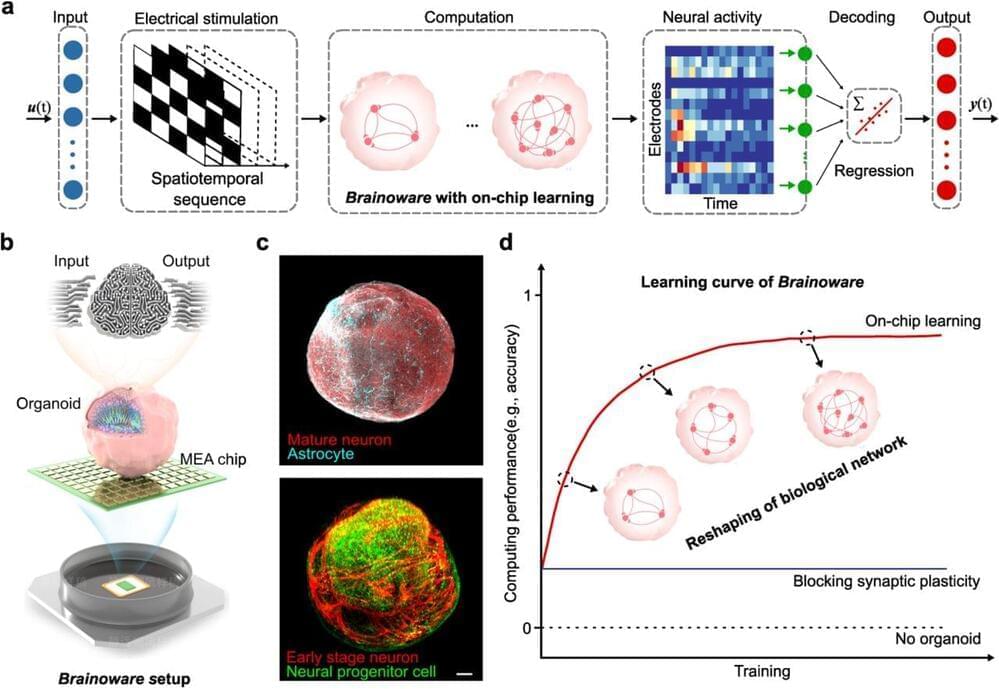
Brain-inspired hardware emulates the structure and working principles of a biological brain and may address the hardware bottleneck for fast-growing artificial intelligence (AI). Current brain-inspired silicon chips are promising but still limit their power to fully mimic brain function for AI computing. Here, we develop Brainoware, living AI hardware that harnesses the computation power of 3D biological neural networks in a brain organoid. Brain-like 3D in vitro cultures compute by receiving and sending information via a multielectrode array. Applying spatiotemporal electrical stimulation, this approach not only exhibits nonlinear dynamics and fading memory properties but also learns from training data. Further experiments demonstrate real-world applications in solving non-linear equations. This approach may provide new insights into AI hardware.
Artificial intelligence (AI) is reshaping the future of human life across various real-world fields such as industry, medicine, society, and education1. The remarkable success of AI has been largely driven by the rise of artificial neural networks (ANNs), which process vast numbers of real-world datasets (big data) using silicon computing chips 2, 3. However, current AI hardware keeps AI from reaching its full potential since training ANNs on current computing hardware produces massive heat and is heavily time-consuming and energy-consuming 4– 6, significantly limiting the scale, speed, and efficiency of ANNs. Moreover, current AI hardware is approaching its theoretical limit and dramatically decreasing its development no longer following ‘Moore’s law’7, 8, and facing challenges stemming from the physical separation of data from data-processing units known as the ‘von Neumann bottleneck’9, 10. Thus, AI needs a hardware revolution8, 11.
A breakthrough in AI hardware may be inspired by the structure and function of a human brain, which has a remarkably efficient ability, known as natural intelligence (NI), to process and learn from spatiotemporal information. For example, a human brain forms a 3D living complex biological network of about 200 billion cells linked to one another via hundreds of trillions of nanometer-sized synapses12, 13. Their high efficiency renders a human brain to be ideal hardware for AI. Indeed, a typical human brain expands a power of about 20 watts, while current AI hardware consumes about 8 million watts to drive a comparative ANN6. Moreover, the human brain could effectively process and learn information from noisy data with minimal training cost by neuronal plasticity and neurogenesis,14, 15 avoiding the huge energy consumption in doing the same job by current high precision computing approaches12, 13.