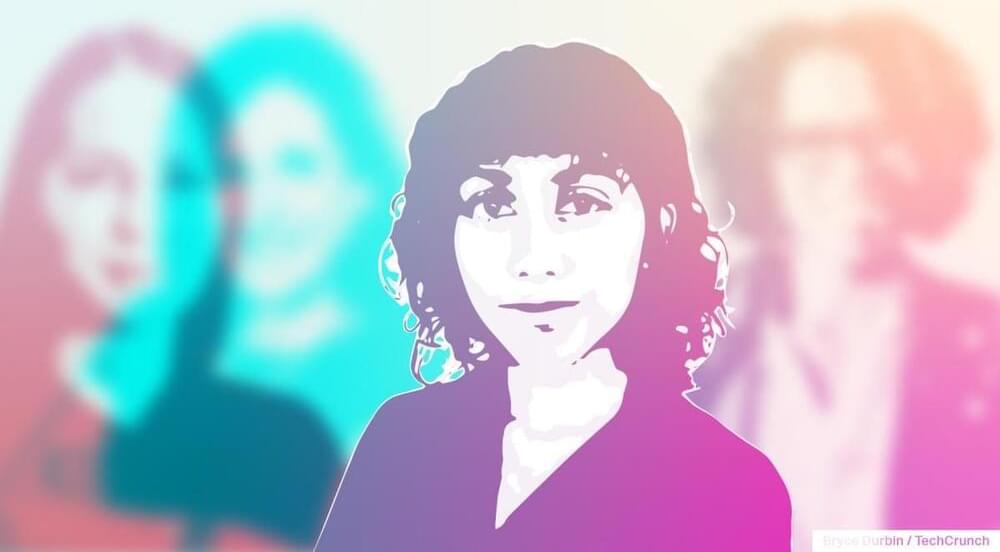
To give AI-focused women academics and others their well-deserved — and overdue — time in the spotlight, TechCrunch is launching a series of interviews focusing on remarkable women who’ve contributed to the AI revolution. We’ll publish several pieces throughout the year as the AI boom continues, highlighting key work that often goes unrecognized. Read more profiles here.
Irene Solaiman began her career in AI as a researcher and public policy manager at OpenAI, where she led a new approach to the release of GPT-2, a predecessor to ChatGPT. After serving as an AI policy manager at Zillow for nearly a year, she joined Hugging Face as the head of global policy. Her responsibilities there range from building and leading company AI policy globally to conducting socio-technical research.
Solaiman also advises the Institute of Electrical and Electronics Engineers (IEEE), the professional association for electronics engineering, on AI issues, and is a recognized AI expert at the intergovernmental Organization for Economic Co-operation and Development (OECD).