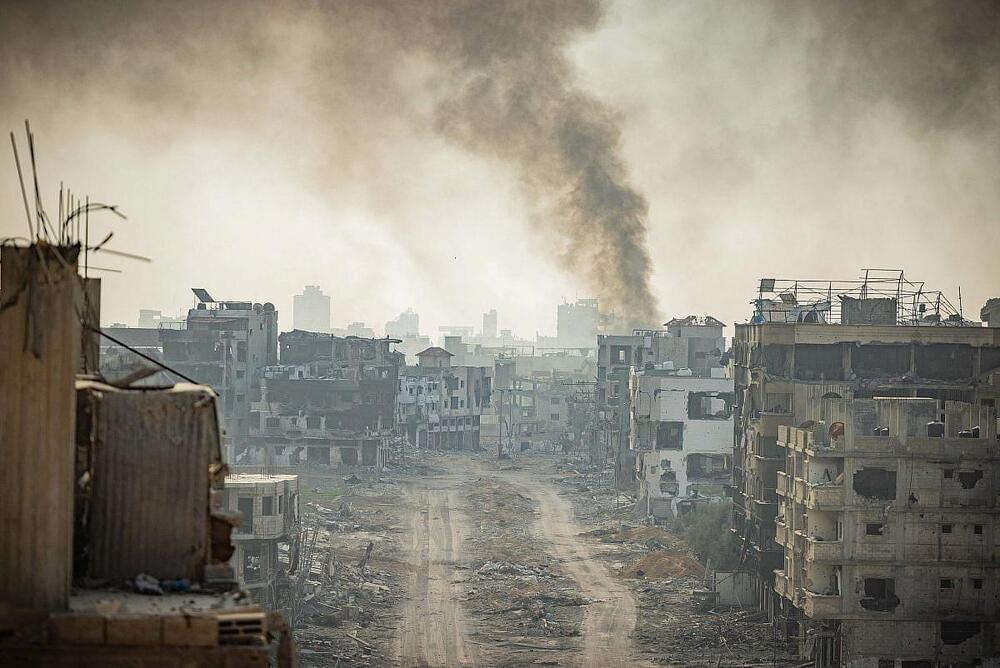
In 2021, a book titled “The Human-Machine Team: How to Create Synergy Between Human and Artificial Intelligence That Will Revolutionize Our World” was released in English under the pen name “Brigadier General Y.S.” In it, the author — a man who we confirmed to be the current commander of the elite Israeli intelligence unit 8,200 — makes the case for designing a special machine that could rapidly process massive amounts of data to generate thousands of potential “targets” for military strikes in the heat of a war. Such technology, he writes, would resolve what he described as a “human bottleneck for both locating the new targets and decision-making to approve the targets.”
Such a machine, it turns out, actually exists. A new investigation by +972 Magazine and Local Call reveals that the Israeli army has developed an artificial intelligence-based program known as “Lavender,” unveiled here for the first time. According to six Israeli intelligence officers, who have all served in the army during the current war on the Gaza Strip and had first-hand involvement with the use of AI to generate targets for assassination, Lavender has played a central role in the unprecedented bombing of Palestinians, especially during the early stages of the war. In fact, according to the sources, its influence on the military’s operations was such that they essentially treated the outputs of the AI machine “as if it were a human decision.”
Formally, the Lavender system is designed to mark all suspected operatives in the military wings of Hamas and Palestinian Islamic Jihad (PIJ), including low-ranking ones, as potential bombing targets. The sources told +972 and Local Call that, during the first weeks of the war, the army almost completely relied on Lavender, which clocked as many as 37,000 Palestinians as suspected militants — and their homes — for possible air strikes.